
BUS308 Week 5 – Lecture 2
A Different View: Confidence Intervals
Expected Outcomes
After reading this lecture, the student should be familiar with:
1. How to construct a confidence interval for a mean.
2. How to interpret a confidence interval for a mean.
3. How to construct a confidence interval for mean differences.
4. How to interpret a mean difference confidence interval.
Overview
This week we are changing focus again. From looking at differences and relationships, we are going to look at considering what we can take from our findings – broadening, so to speak, our interpretation of what we have found out.
Our focus will take us to two distinctly different views of the findings. The first topic will be confidence intervals. We saw something similar to these when we found which pairs of means different with our ANOVA outcomes. One of the most commonly seen example of confidence intervals comes with opinion polling data. We often see a result such as 56% of the population approves of the idea with a margin of error of +/- 3%. This means that the real percentage if everyone were asked would lie between 53 and 59% – the confidence interval. The second will be something called Effect Size, a way of examining how practically significant a finding is. Effect sizes will be discussed in the third lecture for this week.
Confidence Intervals
One thing we might have noticed during this class is that different samples from the same population (technically, with replacement, putting previously selected individuals back into the pool for a chance of being selected again), give us different results. The sample used in the lectures was different from the sample used in the homework assignments, at least with the salary and compa-ratio values. Yet, even with these sample differences, the outcomes of the statistical tests were the same – we rejected or not rejected the same hypothesis statements with our slightly different values.
So, many want to know the range of values that would could be a population’s mean based on the sample results, particularly the variation present in the sample. A confidence interval can provide us with this information. This is basically a range of values that show us the possible sampling error in our results. When we construct them, we are able to specify the level of confidence we desire that the interval will contain the actual population mean – 90%, 95%, 99%, etc.
Confidence intervals often provide the added information and comfort about estimates of population parameter values that the single point estimates lack. Since the one thing we do know about a statistic generated from a sample is that it will not exactly equal the population
parameter, we can use a confidence interval to get a better feel for the range of values that might be the actual population parameter. They also give us an indication of how much variation exists in the data set – the larger the range (at the same confidence level), the more variation within the sample data set – and the less representative the mean would be. We are going to look at two different kinds of confidence intervals this week – intervals for a one sample mean and intervals for the differences between the means of two samples.
One Sample Confidence Interval for the mean
A confidence interval is simply a range of values that could contain the actual population parameter of interest. It is centered on the sample mean, and uses the variation in the sample to estimate a range of possible values. To construct a confidence interval, we use several pieces of information from the sample, and the confidence level we want.
From the sample we use the mean, standard deviation, and size. To get the confidence level – a desired probability, usually set at 95%, that the interval does, in fact, contain the population mean – we use a related t value.
One-Sample Intervals
Example. Confidence intervals can be generated manually or we can use the Excel Confidence.T function found in the fx or Formulas statistical list. To compare the two, we will construct a 95% confidence interval for the female compa-ratio. Our sample mean value is 1.069, the standard deviation is 0.70, and the sample size is 25 (from week 1 material).
Our confidence interval formula is mean +/- t* standard error of the mean, which is the same as: mean +/- t * stdev/sqrt(sample size).
Once we determine the confidence level we want, we use the associated 2-tail t value to achieve it. The t-value is found with the fx function t.inv.2t (Prob, df). For a 95% confidence interval, we would use t.inv.2t(0.05, 24), this equals 2.064 (rounded). Remember that the df for a t is n-1, so for a sample of 25 it equals 24.
We now have all the information we need to construct a 95% confidence interval for the female compa-ratio mean:
CI = mean +/- t * stdev/sqrt(sample size) = 1.069 +/- 2.064*0.070/sqrt(25) = 1.069 +/- 0.029.
This is typically written as 1.04 to 1.098. Note: the standard deviation divided by the square root of the sample size is called the standard error of the mean, and is the variation measure of the sample used in several statistical tests, including the t-test and confidence intervals.
Now, let’s look at how Excel can help us with this. Excel has two confidence interval functions, one based on the z distribution (often used for large samples) and another based on the t distribution. The t-based interval is becoming more and more of the standard with the wide availability of Excel and other statistical programs. So, we will use it.
The Fx (or Formulas) function Confidence.t will give us the margin of error (the +/- term) for any desired interval. Here is a screenshot of this tool, with our Female Compa-ratio values filled in. Note that our value from above (0.029 rounded) is shown even without hitting OK.
The associated 95% CI for males is 1.022 to 1.091. Note that the ranges overlap. The female range of 1.04 to 1.098 (with higher endpoint values than the male range) and the male range of 1.022 to 1.091(with lower endpoint values) overlap quite a bit: between 1.04 to 1.091 of each range is common to both. How do we interpret this overlap? We cannot immediately say an overlap means the two means might be equal. We will look at the reason for this after looking at confidence intervals for differences below.
In the meantime, there are a couple of guidelines we can use.
• In general, the more overlap, the less significant the difference is.
• If the bottom quarter of the higher value range overlaps with the top quarter of the lower value range, the means would be found to be significantly different at the alpha = 0.05 level.
• If the bottom value of the higher value range just touches the top value of the lower valuer range, the means would be found to be significantly different at the .01 alpha level.
• If the ranges do not overlap at all, the means are significantly different at an alpha less than .01 level.
• If the intervals overlap more than at the extreme quarters, the means are not significantly different at the alpha = .05 level.
Since these CIs overlap more than a single quarter of their ranges, our interpretation would be that they are not significantly different at the alpha = .05 level. This is what we found in week 1 when we performed the t-test for mean difference.
The Confidence Interval for mean differences.
When comparing multiple samples, it is always best to use all the possible information in a single test or procedure. The same is true for confidence intervals. Rather than calculate 2 CIs to determine if means differ, we can use a single Confidence Interval for the difference. If we
are interested in seeing if sample means could be equal, we look to see if the interval for their difference contains 0 or not. If so, then the means could be the same; if not, then the means must be significantly different. This interpretation of the interval is the same as we used with the ANOVA intervals in week 3.
The formula for the mean difference confidence interval is mean difference +/- t*standard error. The standard error for the difference of two populations is found by adding the variance/sample size (which is the standard error squared) for each and taking the square root. For our compa-ratio data set we have the following values:
Female mean = 1.069 Male mean = 1.056 t = t.inv.2t(0.05, 48) = 2.106
Female Stdev = 0.070 Male Stdev = 0.084 Sample size = 50, df = 48
Female variance = 0.070^2 = 0.0049 Male variance = 0.084^2 = 0.0071
Standard error = sqrt(Variance (female)/25) + Variance (male)/25) =
Sqrt(0.0049/25 + 0.0071/25) = sqrt(.000196 + 000284) =0.0219.
This gives us a 95% confidence interval for the difference equaling:
(1.069-1.056)) +/- 2.011 * 0.0219 = 0.013 +/- 0.044 = -0.031 to 0.057.
Since this confidence interval does contain 0, we are 95% confident that the male and female compa-ratio means are are likely equal – which is the same result we got from our 2-sample t-test in week 2. We also now have a sense of how much variation exists in our measures. One interpretation of these two intervals is that the averages are clearly similar rather than just barely the same.
Side note: The “+/- t* SE” term is often called the margin of error. We most often hear this phrase in conjunction with opinion polls – particularly political polls, “candidate A has 43% approval rating with a margin of error of 3.5%. While we do not deal with proportions in the class, they are calculated the same as an empirical probability – number of positive replies divided by the sample size. The construction of these margins or confidences is conceptually the same – a t-value and a standard error of the proportion based on the sample size and results.
Now, let’s go back and look at why two individual confidence intervals do not provide the same information on differences as a single CI for differences. The reason lies in the statistic used for our confidence level. The male and female individual mean confidence intervals used a t value of 2.064 while the CI for the difference used the smaller value of 2.011, due to the larger sample size. This difference in the t value between individual and difference intervals is the reason why the individual intervals cannot be used for a direct interpretation of differences; they are a bit too large for the combined data set.
Summary
Confidence intervals give us added insight into what our sample results are saying about the population. By developing a confidence interval around a mean estimate we can see the range of values that are reasonable estimates for the actual parameter we are trying to estimate. By looking at the spread within the interval, we can get a feeling for how much variation exists in the population (by using the variation within the sample).
Confidence intervals can give us a feeling about the differences between mean estimates. Both are built the same way: our estimate (sample result or difference between means) +/- t times the standard error (the standard deviation divided by the square root of the sample size). The t value is obtained using our desired confidence level and the degrees of freedom associated with our estimate.
Individual estimates of means can be used to get a feel for the difference between population means but they are harder to interpret precisely than using the mean difference confidence interval.
Please ask your instructor if you have any questions about this material.
When you have finished with this lecture, please respond to Discussion Thread 2 for this week with your initial response and responses to others over a couple of days before reading the third lecture for the week.
What Students Are Saying About Us
.......... Customer ID: 12*** | Rating: ⭐⭐⭐⭐⭐"Honestly, I was afraid to send my paper to you, but you proved you are a trustworthy service. My essay was done in less than a day, and I received a brilliant piece. I didn’t even believe it was my essay at first 🙂 Great job, thank you!"
.......... Customer ID: 11***| Rating: ⭐⭐⭐⭐⭐
"This company is the best there is. They saved me so many times, I cannot even keep count. Now I recommend it to all my friends, and none of them have complained about it. The writers here are excellent."
"Order a custom Paper on Similar Assignment at essayfount.com! No Plagiarism! Enjoy 20% Discount!"
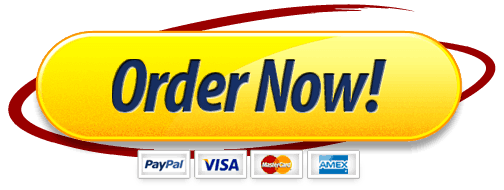