
Assume the role of a research coordinator for the senior research team that published this paper. They have asked you to work with the information technology (IT) department to design a unique research database that can be used to do further health sciences research on this topic. In preparation for your first meeting with IT, you are asked to think about the kinds of data you would like in the research database, the source systems that should feed that data, and the way that data should be organized in the research database.
In your paper,
Explain the rationale for the different types of data that should populate the new research database.
Assess the different source feeder systems that should supply this data to the research database.
Construct a strategy for how the scientist on the research team cannot utilize the relational research database to increase their opportunity for research funding.
Create the basic design for the research DBMS that you propose including the main tables in the database, using Microsoft Word Shapes or Microsoft PowerPoint functionality.
Format your design as a figure and embed the design your paper.
Describe the fields that you would include in each of the tables in your DBMS drawing.
The Developing a Research Data Warehouse paper
Mu
RESEARCH ARTICLE Open Access
Dissecting genetic factors affecting phenylephrine infusion rates during anesthesia: a genome-wide association study employing EHR data Yanfei Zhang1, S. Mark Poler2, Jiang Li3, Vida Abedi3, Sarah A. Pendergrass4, Marc S. Williams1 and Ming Ta Michael Lee1,5*
Abstract
Background: The alpha-adrenergic agonist phenylephrine is often used to treat hypotension during anesthesia. In clinical situations, low blood pressure may require prompt intervention by intravenous bolus or infusion. Differences in responsiveness to phenylephrine treatment are commonly observed in clinical practice. Candidate gene studies indicate genetic variants may contribute to this variable response.
Methods: Pharmacological and physiological data were retrospectively extracted from routine clinical anesthetic records. Response to phenylephrine boluses could not be reliably assessed, so infusion rates were used for analysis. Unsupervised k-means clustering was conducted on clean data containing 4130 patients based on phenylephrine infusion rate and blood pressure parameters, to identify potential phenotypic subtypes. Genome-wide association studies (GWAS) were performed against average infusion rates in two cohorts: phase I (n = 1205) and phase II (n = 329). Top genetic variants identified from the meta-analysis were further examined to see if they could differentiate subgroups identified by k-means clustering.
Results: Three subgroups of patients with different response to phenylephrine were clustered and characterized: resistant (high infusion rate yet low mean systolic blood pressure (SBP)), intermediate (low infusion rate and low SBP), and sensitive (low infusion rate with high SBP). Differences among clusters were tabulated to assess for possible confounding influences. Comorbidity hierarchical clustering showed the resistant group had a higher prevalence of confounding factors than the intermediate and sensitive groups although overall prevalence is below 6%. Three loci with P < 1 × 10−6 were associated with phenylephrine infusion rate. Only rs11572377 with P = 6.09 × 10−7, a 3′UTR variant of EDN2, encoding a secretory vasoconstricting peptide, could significantly differentiate resistant from sensitive groups (P = 0.015 and 0.018 for phase I and phase II) or resistant from pooled sensitive and intermediate groups (P = 0.047 and 0.018).
(Continued on next page)
© The Author(s). 2019 Open Access This article is distributed under the terms of the Creative Commons Attribution 4.0 International License (http://creativecommons.org/licenses/by/4.0/), which permits unrestricted use, distribution, and reproduction in any medium, provided you give appropriate credit to the original author(s) and the source, provide a link to the Creative Commons license, and indicate if changes were made. The Creative Commons Public Domain Dedication waiver (http://creativecommons.org/publicdomain/zero/1.0/) applies to the data made available in this article, unless otherwise stated.
* Correspondence: [email protected] 1Genomic Medicine Institute, Geisinger, Danville, PA 17822, USA 5Lab 218, Weis Center for Research, Geisinger, 100 North Academy Ave, Danville 17822-2620, PA, USA Full list of author information is available at the end of the article
Zhang et al. BMC Medicine (2019) 17:168 https://doi.org/10.1186/s12916-019-1405-7
Beyond Big Data to new Biomedical and Health Data Science: moving to next century precision health
http://crossmark.crossref.org/dialog/?doi=10.1186/s12916-019-1405-7&domain=pdf
http://orcid.org/0000-0003-2510-8977
http://creativecommons.org/licenses/by/4.0/
http://creativecommons.org/publicdomain/zero/1.0/
mailto:[email protected]
(Continued from previous page)
Conclusions: Retrospective analysis of electronic anesthetic records data coupled with the genetic data identified genetic variants contributing to variable sensitivity to phenylephrine infusion during anesthesia. Although the identified top gene, EDN2, has robust biological relevance to vasoconstriction by binding to endothelin type A (ETA) receptors on arterial smooth muscle cells, further functional as well as replication studies are necessary to confirm this association.
Keywords: Phenylephrine, Anesthesia, Hypotension, GWAS, EDN2, Electronic health records (EHR), Unsupervised clustering, Vasoconstriction, Pharmacogenomics,
Background Phenylephrine is a very selective α1-adrenergic receptor agonist frequently used for treatment and prevention of hypotension during anesthesia or critical care. It is one of the most commonly used drugs for treatment of in- traoperative hypotension [1]. Phenylephrine infusions are used to sustain blood pressure at clinically acceptable levels during anesthesia. Inter-individual differences in response to phenylephrine have been frequently ob- served in clinical practice [2–4]. Patients’ response to phenylephrine may vary depending upon age, co-mor- bidities (e.g., cardiovascular diseases), concurrent medi- cations, and anesthetic status. Genetic factors may also contribute to this response variability. While some work has been done looking at genetic in-
fluence of ADRB2 on hemodynamic response [5, 6], very little work on phenylephrine response has been pub- lished beyond some limited candidate gene studies. Al- though it functions as a selective α1-adrenergic receptor agonist, phenylephrine has a moderate β-agonist activity at higher doses [7, 8]. Several candidate gene studies have found that individuals carrying the Ile164 allele in ADRB2, encoding the β2 adrenoreceptor, had a much higher sensitivity to phenylephrine than non-carriers [9]. The Arg16 allele alone or Arg16-Gln27-Thr164-Arg175- Gly351 haplotype was also associated with higher phenylephrine sensitivity [10]. However, this association could not be replicated in a cohort of patients under spinal anesthesia for cesarean delivery and the Arg16 carriers actually required more phenylephrine than non- carriers [11]. The association between 34 single nucleo- tide polymorphisms (SNP) in ADRA1B, encoding α1 ad- renergic receptor 1B subtype, and phenylephrine response was evaluated [12]. rs10070745 was signifi- cantly associated with response to this vasoconstrictor only in patients with African but not European ancestry [12]. There was no report of significant impact of genetic variants from ADRA1A, encoding α1 adrenergic receptor 1A subtype, on phenylephrine response. Although all these candidate gene studies showed some promising connection between pharmacodynamic genes and drug response, the significance of the association has been limited by sample size, number of interrogated genetic
variants, definition of responsiveness (response or non- response as a binary trait), magnitude of variation in re- sponse (quantitative trait), mixture of vasopressors, and hypotheses. Genome-wide association studies (GWAS) employ large patient cohorts and subsequent fine-map- ping techniques that are hypothesis-agnostic and thus not limited to preconceived ideas of the genes involved in the phenotype based on prior knowledge. In clinical situations, phenylephrine is most often ad-
ministered as boluses, less often by infusion. An immedi- ate increase of BP after a bolus, usually prompt in onset and lasting for minutes, could be used to evaluate re- sponsiveness to phenylephrine. This requires frequent BP measurements during the effect window, a require- ment not satisfied by the available research data ex- tracted from clinical anesthesia records. Alternatively, intravenous (IV) infusion rate (dose) could be used to estimate phenylephrine sensitivity. Infusion rates are de- termined empirically by clinicians, adjusted dynamically as needed by changing conditions, and subject to numer- ous unaccounted determinants (e.g., blood loss and vol- ume replacement, concurrent drug effects, and surgical events and requirements) to maintain situationally ap- propriate blood pressures. Infusion rates can be quite variable while being titrated to effect, then rather stable for long periods after completion of initial adjustments. With a large patient cohort, individual variations are ex- pected to be randomly distributed relative to the genetic variations. Geisinger is an integrated healthcare provider located
in central and northeastern Pennsylvania and southern New Jersey, having an electronic health record (EHR) system which captures a median of 14 years of compre- hensive electronic records for participants in the MyCode® Community Health Initiative (MyCode) which include but are not limited to patients’ demographic fea- tures, primary diagnoses and co-morbidities, laboratory measurements, prescriptions, vital signs, and surgical procedure logs [13]. Intraoperative electronic anesthesia records have been active since July 2012. Whole exome sequencing and genome-wide genotyping data are avail- able for more than 92,000 MyCode participants to date [13, 14]. The coupled genotype and longitudinal
Zhang et al. BMC Medicine (2019) 17:168 Page 2 of 14
phenotype data provide unique opportunities for us to conduct GWAS based on this “real world” clinical data and to yield clinically relevant insights [15]. In this study, we present the results of the first GWAS
for phenylephrine response defined by phenylephrine in- fusion rate using real-world EHR data.
Methods Study cohort and institutional review board This study population consisted of 12,688 individuals with available electronic anesthesia records from the Geisinger de-identified EHR database who met the in- clusion criteria described in more detail below. We re- ceived an exemption from the institutional review board (IRB) for a non-human subject study as all the EHR data were de-identified. For the genetic study, we received ap- proval from the IRB at Geisinger and the MyCode Gov- erning Board. All MyCode participants provide a consent that allows their clinical and genomic data to be used for health-related research. Details of the consent- ing process are described elsewhere [9]. Approximately 40% of these patients with available anesthesia records were MyCode participants with genetic data.
EHR data extraction In Geisinger clinical practice, as at many other institu- tions, phenylephrine is typically the first-line vasopressor for treatment of hypotension during anesthesia. How- ever, there are no standardized phenylephrine infusion guidelines, BP targets, or practices for cases in this op- portunistic cohort. Pharmacy prepares standard concen- trations of phenylephrine for infusions. Choice and management of phenylephrine infusions was entirely at the discretion of anesthesia clinicians. General anesthesia predominates for surgeries at Geisinger, although spinal and regional anesthetics are employed with and without general anesthesia. Data de-identification and extraction were conducted by Geisinger’s Phenomic Analytics & Clinical Data Core. Patient information of those who had electronic anesthesia records between July 2012 and November 2016 excluding cesarean sections and trauma cases was obtained. The following de-identified data ele- ments were requested: surgery duration; anesthetic agents and other intraoperative drugs, doses and total quantity of phenylephrine; start and end time of each phenylephrine infusion; phenylephrine infusion rates; blood pressures; age and weight at the time of surgery; International Classification of Disease v. 9 (ICD-9) coded diagnoses; and demographics. The extracted anesthetic record data originated from multiple Geisinger out- patient and inpatient sites distributed across a large geo- graphical area. There was no selection for patient characteristics, types of procedures, surgical depart- ments, or anesthetic techniques.
EHR data mining and modeling Quality control (QC) and sample filtration Analyses employed SBP because it is the most consist- ently present blood pressure parameter and has larger changes in response to phenylephrine treatment than mean artery pressure (MAP) or DBP, though MAP may be a more robust physiological measurement and better correlated between invasive and non-invasive BP mea- surements. We removed obvious errors in the data by identifying values that were not representative of the measurement, such as implausible values (e.g., BMI = 1000), and extreme SBP values (SBP 200 mmHg) as they were less likely to be caused by genetic factors, but more likely to be data errors or artifacts, such as arterial line flushes, disconnections, or physiological extremis. Patients who had ephedrine and/ or phenylephrine boluses during phenylephrine infusion, patients who had short infusion periods (< 10 min), or few blood pressure measurements during the infusion interval (< 3 data points) were also excluded from fur- ther analysis. Median count for SBP measurements per patient is 16. These quality-checked data were then used for data modeling (Fig. 1).
Data modeling Empirical observation discerns three types of response to phenylephrine infusion: normal or high BP with low infusion rate (“sensitive”), correction of severe hypotension but still low BP with low infusion rate (“intermediate”), and ameliorated yet still low BP requir- ing high infusion rate (“resistant”). A “fourth” hypothet- ical cluster having high BP at high infusion rates is not represented in the data because clinicians rarely need to use phenylephrine to increase already normal or high blood pressures. k-means clustering was selected as our unsupervised machine learning algorithm as it is simple and computationally efficient to solve known cluster is- sues within large datasets. To reflect the clinical observa- tions, cluster numbers (k = 2 and 3) were evaluated using three key features related to phenylephrine re- sponse: mean and standard deviation (SD) of systolic blood pressure (SBP) during infusion period and average infusion rate of phenylephrine. We also evaluated the k- means clustering on a broader set of features including weight, age, and infusion duration. Data standardization and k-means clustering were performed using Python (2.7.14) scikit-learn library (0.19.1).
Clinical feature analysis To determine whether there were statistical differences in clinical features between clustering derived subgroups, chi- square tests were used for categorical data, and one-way ANOVA was used for continuous data. These statistical analyses were performed using GraphPad Prism (7.04). The
Zhang et al. BMC Medicine (2019) 17:168 Page 3 of 14
P value 0.7 were included in the analysis. Samples with genotyping rate below 95% were
excluded. SNPs with < 99% call rate, minor allele fre- quency < 1%, and significant deviation from Hardy- Weinberg equilibrium (P < 10−7) were removed. At last, there were 4,929,806 SNPs in phase I and 2,978,370 SNPs in phase II included in the analysis. One of paired of individuals with first- or second-degree relatedness, as determined by IBD analysis were removed from analysis. PLINK 1.9 [17] was used for genotypic quality control.
Association tests and the meta-analysis The flowchart and sample size of phase I and phase II cohorts are shown in Fig. 1. Ninety-seven of the 174 pa- tients, who had multiple surgeries partitioned to differ- ent clusters for different procedures by k-means clustering, were removed from further analyses. The mean values of the SBP, age, and weight for the other 77 patients who had concordant cluster associations from multiple surgeries were used to avoid non-independent measurements in the association test. Finally, 1574 pa- tients were subject to genetic analyses. A linear regres- sion model for average infusion rate with full set of covariates without interaction terms was conducted first to decide which covariates to adjust in the genetic asso- ciation test. Covariates that were evaluated included age, sex, weight, mean SBP, SD of SBP, anesthesia type, and comorbidities that may affect the pharmacokinetics and blood pressure, including diabetes, hypertension, lipid metabolism disorders, overweight and obesity, ischemic
Fig. 1 EHR data mining and GWAS pipeline. A total of 14,213 distinct anesthesia episodes that had phenylephrine infusions were identified from the EHR data. 4033 patients were included for k-means clustering after applying exclusion criteria such as a short infusion period (< 10 min), concurrent ephedrine and/or phenylephrine bolus injections during the infusion period, blood pressure values that were not consistently obtained by either invasive or non-invasive measurement, a limited number of SBP measurements, implausible infusion rate units, and missing body weight. Linear regression model for phenylephrine infusion rate was selected for association testing under an additive genetic mode followed by a fix effect inverse variance meta-analyses of phase I and phase II datasets. Top associated loci were further tested by comparing resistant versus sensitive or resistant versus pooled sensitive and intermediate groups. SBP, systolic blood pressure
Zhang et al. BMC Medicine (2019) 17:168 Page 4 of 14
heart disease, heart failure, and cardiac dysrhythmias. Only age, sex, weight, mean of SBP, and SD of SBP were significantly associated with the infusion rate (Add- itional file 1: Table S1). Thus, they were included to- gether with first six principal components (PCs) as covariates in GWAS tests. PLINK 1.9 was used to perform the genetic association
analyses. A linear regression model was adopted for GWAS on the average infusion rate adjusted for the sig- nificant covariates and the first six principal components in phase I and phase II cohorts followed by a fixed effect inverse variance meta-analysis by METAL [18]. The lead SNPs were further evaluated in the case-control associ- ation test in subgroups identified by k-means clustering using logistic models adjusting for age, sex, body weight, and 6 PCs. Mean and SD of BP were used in the deter- mination of clusters and thus were not included in the model. The resistant group was compared to the sensi- tive group alone, or the pooled intermediate + sensitive groups. GTEx [19], Ensemble VEP [20], USCS genome
browser [21], and STRING [22] were used for eQTL, variant annotation, and other functional genomics quer- ies. Power test for the top hit with strong biological rele- vance was conducted using Quanto.
Results A total of 14,213 distinct anesthesia episodes that had phenylephrine infusions were identified from the EHR data. Of these, 9895 were excluded during data cleaning due to a short infusion period (< 10 min), concurrent confounding ephedrine and/or phenylephrine bolus in- jections during the infusion period, blood pressure values that were not exclusively obtained by either inva- sive or non-invasive measurements, fewer than three SBP measurements during phenylephrine infusion, im- plausible infusion rate units, and missing body weight (Fig. 1). A total of 4033 unique patients were included in the analyses. The demographic features are listed in Table 1. The average age at the time of surgery was 62.4 years old. Most of the patients are of European ancestry (97.7%) and had surgeries performed under general anesthesia.
Three sub-groups were identified for phenylephrine responsiveness k-means clustering was employed as our unsupervised machine learning approach to categorize the response to phenylephrine, for 2 or 3 clusters evaluated on three key features related to phenylephrine response: average infu- sion rate (mcg/min), mean SBP (mmHg), and SD of SBP. SD of SBP reflects the blood pressure variability during the infusion period. When two clusters were con- sidered (k = 2), the separation was made by blood
pressures but not by average infusion rate (Additional file 2: Figure S1A). In a two-cluster model, 36% of the pa- tients were classified as poor responders (Additional file 2: Figure S1B), which was higher than clinically ob- served empirical rate of 10~20%. When three clusters were evaluated (k = 3), patients were classified into three categories that can be described as intermediate re- sponders (n = 2236, 56%), having low mean SBP under low infusion rate of phenylephrine; resistant responders (n = 531, 13%), requiring higher infusion rate to main- tain low mean SBP; and sensitive responders (n = 1266, 31%), having higher mean SBP with low infusion rates (Fig. 2a and b). Ninety-seven patients were dropped from the analysis due to inconsistent clustering when comparing the results from two or more anesthesia epi- sodes for the same individual. There were no clear boundaries between clusters when average infusion rate was plotted against mean SBP (Fig. 2a), indicating the response is not a discrete trait; however, the degree of overlap is modest, supporting the clinical observations. We have evaluated k-means clustering on other feature
combinations. However, we did not observe more defini- tive or distinct clusters. In contrast, the boundaries be- came less definitive with the number of features increasing, especially for the resistant group, which is the most clinically interesting (Additional file 3 Figure S2).
Clinical features among sub-groups of distinct phenylephrine responsiveness Since responsiveness can be affected by multiple genetic or non-genetic factors, the clinical features in each sub- group were further compared by chi-square test or one- way ANOVA for categorical and quantitative features, re- spectively (Fig. 2c and Table 2). The means for average in- fusion rate, SBP, and SD of SBP were significantly different among three clusters. Other than anesthesia type, clinical features such as age, sex, and weight, were signifi- cantly different among these groups (Table 2). The
Table 1 Population demographics
Characteristics Value
Total number of patients 4033
Sex (M, F) 1902, 2131
Age, years (mean ± SD) 62.4 ± 14.4
Race
European ancestry 3941
African ancestry 70
Other 22
Anesthesia type
General 3709
Spinal 163
Others 161
Zhang et al. BMC Medicine (2019) 17:168 Page 5 of 14
resistant group had a lower percentage of female patients (P = 2.17 × 10−10) and higher body weight (P = 0.0016) than the intermediate and the sensitive groups, suggesting that sex and body weight could possibly be predictors of phenylephrine response and should be included as con- founding factors in the association study. One of the key pharmacokinetic parameters, volume of distribution (VD) of a drug, which is the circulation volume for phenyleph- rine infusion, could be significantly affected by body weight. The mean age of sensitive responders was signifi- cantly higher than that of intermediate (P = 1.759 × 10−12) and resistant patients (P = 2.2 × 10−16).
Different comorbidities among sub-groups of phenylephrine responsiveness Comorbidities and the three subgroups of patients with differential response to phenylephrine were clustered and characterized. The resistant group was well sepa- rated from the sensitive and intermediate groups in hier- archical clustering (Fig. 3). The resistant group has a higher prevalence of diabetes (P = 0.00023), heart failure (P = 0.003), chronic kidney disease (P = 0.02), overweight (P = 0.04), and disorders of fluid-electrolyte and acid- base balance (P = 0.04), suggesting more confounding factors and severity of illness in the resistant group.
Fig. 2 k-means clustering based on phenylephrine infusion rate, mean SBP, and SD values of SBP. a Scatter plot of mean SBP against average infusion rate after k-means clustering when k = 3. b Pie chart to show the proportion of each identified sub-group. c Violin plots to show the distribution of individual clinical parameter among three clustered subgroups. X-axis represents the response clusters: resistant, intermediate, and sensitive. Y-axis represents each clinical parameter. SBP, systolic blood pressure; SD, standard deviation
Zhang et al. BMC Medicine (2019) 17:168 Page 6 of 14
Table 2 The clinical features corresponding to the three groups
Resistant Intermediate Sensitive P value*
No. of Patients 531 2236 1266 /
Sex: female, N (%) 239 (45.0) 1131 (50.6) 761 (60.1) < 0.0001
Anesthesia type %
General 421 (79.3) 1806 (80.9) 1068 (84.3)
Spinal 21 (3.9) 99 (4.4) 43 (3.4) 0.0354
Others 89 (16.8) 331 (14.7) 155 (12.3)
Age at surgery (years) 62.4 ± 13.9 59.5 ± 14.7 67.4 ± 12.5 < 0.0001
Body weight (kg) 94.7 ± 27.6 88.6 ± 23.0 85.4 ± 22.1 < 0.0001
Avg. infusion rate (mcg/min) 76.2 ± 22.4 32.1 ± 11.8 32.4 ± 12.3 < 0.0001
Mean SBP (mmHg) 108.0 ± 11.0 105.4 ± 8.7 123.9 ± 10.9 < 0.0001
SD of SBP 15.0 ± 6.4 10.0 ± 3.7 19.9 ± 7.3 < 0.0001
*P value was calculated from chi-square test for categorical variables, and one-way ANOVA for continuous variables. P value < 0.00625 was considered significant
Fig. 3 Heatmap of comorbidity prevalence in the three subgroups identified by k-means clustering. X-axis represents the subgroups; Y-axis represents the comorbidities. Hierarchical clustering was performed on both X- and Y-axis using Euclidean distance and average linkage method. The redder, the higher prevalence. The greener, the lower prevalence. The prevalence of disease across the subgroups was evaluated by chi- square test. The red triangle represents P < 0.001; the red dot represents P < 0.01; the green dot represents P < 0.05
Zhang et al. BMC Medicine (2019) 17:168 Page 7 of 14
These could reflect physiological alterations or more clinically significant compromise in these patients, re- quiring more aggressive treatment of hypotension. The overall calculated comorbidity prevalence was low (< 6%) across all three subgroups. This might explain why co- morbidities were not significant covariates in the full model on phenylephrine infusion rate (Additional file 1: Table S1).
Top associations with phenylephrine infusion rate All patients included in this GWAS have genetically verified European ancestry, a characteristic of the Gei- singer regional population that may not be generalizable. We applied a linear regression model to identify the po- tential confounding factors related to average infusion rate (Additional file 1: Table S1). Patients’ age at surgery, sex, and body weight were considered as the covariates in all subsequent association testing. No genome-wide significant loci were identified in either the phase I or phase II cohorts. Twelve independent loci with suggest- ive significance (P < 10−5) were identified to be associ- ated with the phenylephrine average infusion rate after a
meta-analysis. Figure 4a and b illustrate the Manhattan plot and QQ plot for the meta-analysis. Table 3 lists the lead SNP in each locus after a LD-based clumping of the summary statistics. The most significantly associated SNP was rs2069661, flanking the bidirectional genes, F2RL2 and IQGAP2. This SNP is in complete linkage disequilibrium (LD) with rs193230021 and rs116836657, both of which are located at 3′UTR for F2RL2. rs77080086, the second top hit, is an intronic variant in PDE4B that encodes an enzyme that specifically hydro- lyzes cAMP, a critical step in the β-adrenergic receptors signaling [23]. The lead SNP is in complete LD with rs75398902 (A112G), a missense SNP, which is possibly damaging as predicted by PolyPhen (score of 0.506). This mutation may affect the alpha-helix stability of the functional domain of the enzyme [24]. The third top SNP, rs11572377, located at the 3′UTR of EDN2, en- codes endothelin-2, a secretory vasoconstrictive peptide which causes vasoconstrictions by tightly binding to smooth muscle ETA receptors [25]. We failed to replicate the previously reported associ-
ation between Thr164Ile (rs1800888, OR = 0.268, P =
A
C D E
B
Fig. 4 Meta-analysis and subsequent analyses on top hits. a Manhattan plot and b Q-Q plot of the meta-analysis for phenylephrine average infusion rate. Top loci with P < 10–6 were labeled. Genome inflation factor ƛ was 0.979. c Regional association for EDN2 in meta-analyses for phenylephrine infusion rate. 800 kb flanking the genomic region of the lead SNP, marked as purple diamond, were illustrated. d Boxplot of average infusion rate against the rs11572377 genotypes in phase I and phase II cohorts. Raw P value refers to allelic association under additive model of linear regression adjusted for the corresponding covariates. e Protein-protein interaction network for EDN2 identified by STRING. The nodes and edges respectively represent encoded proteins and evidence-based functional interaction derived from a combined score which was computed by combining the probabilities from up to 7 different resources and corrected for the probability of randomly observing an interaction. Only high confidence interactions were shown here (interaction score ≥ 0.9). Phenylephrine node was superimposed to the existing interactive plot. A solid line was created between phenylephrine and ADRA1B or phenylephrine and ADRA1D because of converging evidence from literature. A dashed line was created to show the hypothetical connect between phenylephrine and EDN2 due to lack of solid evidence from literature
Zhang et al. BMC Medicine (2019) 17:168 Page 8 of 14
T a b le
3 Su m m ar y o f le ad
SN P (L D cl u m p ed
) th at
sh o w
su g g es ti ve
as so ci at io n s (P < 10
− 5 ) w it h p h en
yl ep
h rin
e av er ag e in fu si o n ra te
in a m et a- an al ys is
Le ad
SN P
C o o rd in at e (G RC
h 37 )
A 1/ A 2
M A F
Be ta -m
et a (C I9 5)
P- Ph
as eI
P- Ph
as eI I
P- m et a
G en
e U p st re am
g en
e D o w n st re am
g en
e
rs 20 69 66 1
5: 75 91 66 15
A /C
0. 01 72 5
13 .8 5 (8 .6 5~
19 .0 4)
5. 71 E−
06 1. 15 E−
02 1. 80 E−
07 F2 RL 2, IQ G A P2
LO C 10 19 29 10 9
N C RU
PA R
rs 77 08 00 86
1: 66 72 72 50
T/ C
0. 02 46 8
11 .0 7 (6 .8 1 ~ 15 .3 3)
5. 50 E−
07 1. 97 E−
01 3. 51 E−
07 PD
E4 B
LO C 10 19 27 13 9
LO C 10 53 78 77 6
rs 11 57 23 77
1: 41 94 37 95
C /G
0. 01 68 9
13 .0 0 (7 .8 9 ~ 18 .1 1)
5. 44 E−
05 3. 41 E−
03 6. 09 E−
07 ED
N 2
RN A 5S P4 5
rs 14 57 38 72 5
15 :2 58 26 46 1
A /G
0. 01 30 4
14 .4 6 (8 .4 9~
20 .4 2)
2. 50 E−
04 1. 44 E−
03 2. 06 E−
06 LO
C 10 53 70 73 7
A TP 10 A
rs 77 94 85 00
12 :6 02 98 98 8
C /G
0. 01 07 4
− 15 .8 5 (−
22 .3 9~
− 9. 31 )
1. 82 E−
06 2. 29 E−
01 2. 06 E−
06 LO
C 10 09 96 69 6
PG BD
3P 1
rs 14 85 01 69 0
6: 87 01 23 60
A /G
0. 01 43 7
− 13 .8 8 (−
19 .6 1~
− 8. 15 )
2. 33 E−
03 5. 65 E−
05 2. 06 E−
06 N D U FA 5P 9
LO C 10 06 52 96 0
rs 42 34 48 2
3: 13 98 44 24 5
C /G
0. 26 61 0
− 3. 64
(− 5. 15 ~ − 2. 14 )
6. 24 E−
04 4. 11 E−
04 2. 16 E−
06 C LS TN
2 N M N A T3
LO C 10 53 74 13 2
rs 74 40 06 20
7: 12 10 02 70 2
A /T
0. 01 36 4
13 .9 8 (8 .1 3~
19 .8 )
1. 61 E−
06 8. 51 E−
01 2. 83 E−
06 FA M 3C
W N T1 6
C YC
SP 19
rs 20 71 33 6
16 :1 20 61 67 4
A /G
0. 03 25 3
8. 82
(5 .0 4~
12 .5 9)
2. 41 E−
05 8. 37 E−
02 4. 72 E−
06 TN
FR SF 17
LO C 72 99 78
SN X2 9
rs 76 05 41 07
5: 26 67 13 51
T/ C
0. 02 11 3
10 .7 6 (6 .0 9~
15 .4 2)
1. 39 E−
04 1. 27 E−
02 6. 11 E−
06 M SN
P1 C C N B3 P1
rs 16 83 55 59
3: 13 07 49 60 2
A /C
0. 01 46 7
12 .4 7 (6 .9 6~
17 .9 8)
1. 48 E−
05 2. 01 E−
01 9. 19 E−
06 N EK 11
A ST E1
LO C 33 98 74
rs 19 40 55 6
14 :8 86 61 11 2
T/ C
0. 01 91 6
− 10 .9 5 (−
15 .8 0~
− 6. 10 )
9. 94 E−
05 3. 62 E−
02 9. 58 E−
06 KC N K1 0
LO C 10 53 70 61 2
SP A TA 7
Zhang et al. BMC Medicine (2019) 17:168 Page 9 of 14
T a b le
4 Su m m ar y o f th e re su lt s fo r th e to p 3 va ria n ts fr o m
th e as so ci at io n te st in g
SN P
A ve ra g e in fu si o n ra te
Re si st an t vs .s en
si ti ve
Re si st an t vs .( se n si ti ve
+ in te rm
ed ia te )
Ph as e I (N
= 12 05 )
Ph as e II (N
= 32 9)
Ph as e I (N
= 58 9)
Ph as e II (N
= 14 7)
Ph as e I (N
= 12 05 )
Ph as e II (N
= 32 9)
β P
β P
O R
P O R
P O R
P O R
P
rs 20 69 66 1 (F 2R L2 )
13 .7 1 (7 .8 1~
19 .6 )
5. 71 E−
06 14 .3 2 (3 .2 8~
25 .4 )
0. 01 2
3. 50
(1 .5 2~
8. 05 )
3. 19 E−
03 1. 84
(0 .2 6~
13 .1 8)
0. 54 5
3. 90
(1 .9 7~
7. 70 )
9. 12 E−
05 2. 28
(0 .4 3~
12 .2 2)
0. 33 5
Se x
− 4. 05
(− 6. 34 ~ − 1. 76 )
5. 44 E−
04 1. 44
(− 2. 87 ~ 5. 74 )
0. 51 3
0. 50
(0 .3 3~
0. 75 )
7. 97 E−
04 0. 59
(0 .2 5~
1. 35 )
0. 21 0
0. 71
(0 .5 0~
1. 03 )
0. 06 8
1. 00
(0 .4 8~
2. 08 )
0. 99 5
A g e
0. 08
(− 0. 01 ~ 0. 17 )
0. 07 8
0. 16
(− 0. 01 ~ 0. 32 )
0. 06 5
0. 97
(0 .9 6~
0. 99 )
2. 58 E−
03 0. 99
(0 .9 5~
1. 02 )
0. 42 9
1. 01
(0 .9 9~
1. 02 )
0. 33 5
1. 00
(0 .9 7~
1. 03 )
0. 97 4
Bo d y w ei g h t
0. 14
(0 .0 9~
0. 19 )
6. 84 E−
08 0. 18
(0 .0 9~
0. 27 )
1. 19 E−
04 1. 01
(1 .0 0~
1. 02 )
0. 01 9
1. 02
(0 .9 9~
1. 04 )
0. 13 9
1. 01
(1 .0 1~
1. 02 )
4. 40 E−
04 1. 01
(0 .9 97 ~ 1. 03 )
0. 11 2
SN Px W ei g h t
0. 33
(0 .0 8~
0. 57 )
9. 26 E−
03 0. 05
(− 0. 75 ~ 0. 8
What Students Are Saying About Us
.......... Customer ID: 12*** | Rating: ⭐⭐⭐⭐⭐"Honestly, I was afraid to send my paper to you, but you proved you are a trustworthy service. My essay was done in less than a day, and I received a brilliant piece. I didn’t even believe it was my essay at first 🙂 Great job, thank you!"
.......... Customer ID: 11***| Rating: ⭐⭐⭐⭐⭐
"This company is the best there is. They saved me so many times, I cannot even keep count. Now I recommend it to all my friends, and none of them have complained about it. The writers here are excellent."
"Order a custom Paper on Similar Assignment at essayfount.com! No Plagiarism! Enjoy 20% Discount!"
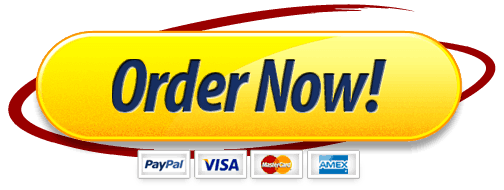